The data quality subject is often neglected, and poor quality business data constitutes a significant cost factor for many online companies. And, although there is much content that claims that the costs of poor data quality are significant, only very few articles are written on how to identify, categorize and measure such costs. In the following article we are going to present how poor quality data can affect your business, why is this happening and how can you prevent wasting time and money due to poor data.
It’s going to be an in-depth geeky research and we advise you to keep your pen and notebook nearby and load yourself with plenty of coffee.
With no further introduction, allow us to present you the main subject we are going to tackle in the following lines:
- Is Poor Data Really Affecting Your Online Business?
- Drowning in Dirty Data? It’s Time to Sink or Swim
- Poor Understanding of the Digital Marketing Environment
- Faulty Methodology Being Used to Collect Digital Marketing Data
- Data That Cannot Be Translated Into Conversion
- Conclusion
Is Poor Data Really Affecting Your Business?
The development of information technology during the last decades has enabled organizations to collect and store enormous amounts of data. However, as the data volumes increase, so does the complexity of managing them. Since larger and more complex information resources are being collected and managed in organizations today, this means that the risk of poor data quality increases, as the studies show. Data management, data governance, data profiling and data warehouse could be the salvation for any business and not just snake oil.
Poor quality data can imply a multitude of negative consequences in a company.
Below you can read just some of them:
- negative economic and social impacts on an organization
- less customer satisfaction
- increased running costs associated
- inefficient decision-making processes
- lower performance (of the business overall but also lowered employee job satisfaction)
- increased operational costs
- difficulties in building trust in the company data
- + many others
In the image below you can easily see that there are not only direct costs we are talking about but hidden costs as well. Therefore, when creating your digital marketing strategy, have in mind the chart below before overlooking the data quality you are working on.
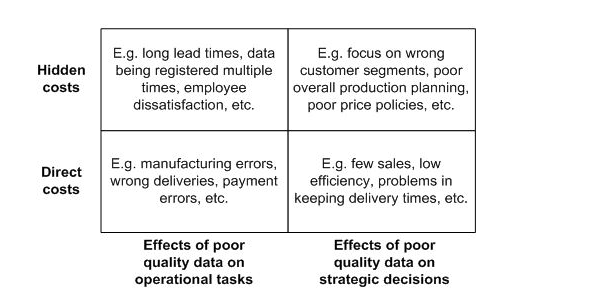
Source: jiem.org
Drowning in Dirty Data? It’s Time to Sink or Swim
95% of new products introduced each year fail.
And yes, this statistic has much to do with the data accuracy; or with the poor marketing data quality. A McKinsey & Company study from a couple of years ago revealed that research is a crucial part of a company’s survival.
80% of top performers did their research, while only 40% of the bottom performers did.
Still, that’s a lot of research done by the bottom performers. Of course, just because they do research doesn’t automatically mean they do good research.
Trying to do research is not enough if you’re not going to be thorough about it.
If you are still not convinced of the importance of having good accurate data, before running any sort of campaign, be it email marketing, SEO or mail automation, take a look at these researched stats below:
- $ 611 bn per year is lost in the US by poorly targeted digital marketing campaigns
- Bad data is the number one cause of CRM system failure
- Organisations typically overestimate the quality of their data and underestimate the cost of errors
- Less than 50% of companies claim to be very confident in the quality of their data
- 33% of projects often fail due to poor data
There are many ways in which research can lead to poor marketing data quality, but let’s look and go in-depth with just 3 particular instances. Taking a close look and understanding them will help us identify how to prevent losing resources (time, money, people). Each of the three instances will be exhaustively detailed below.
- Poor Understanding of The Digital Marketing Environment
- Faulty Methodology Being Used to Collect Digital Marketing Data
- Data That Cannot Be Translated Into Action
1. Poor Understanding of the Digital Marketing Environment
Google Trends is a pocket of gold and you might already know this. Yet, even having this instrument at your disposal, the secret stands in how you use it. Yes, you’ll find a lot of data to help you see what’s trending to stay on top of your competitors. It’s a real-time search data to gauge consumer search behaviors over time. But what happens when you don’t use it properly or when you don’t “read” the the data correctly? Let’s say we are interested in the image search for the digital marketing techniques vs tactics. As we take a look at the screenshot below, we might be inclined to say that the “digital marketing techniques” are of higher interest.
Yet, as mentioned before, I was going for the image search; and even if the techniques seems to be of more interest on the general search, when it comes to image search, I should probably go with tactics. Conducting research is not enough but properly understanding the environment is the key.
On the following line we are going to offer you some nice case studies of poor understanding of the environment and customer needs, based on three dimensions: depth, context and match.
A) No Depth
Companies have certainly come a long way from assuming they know what’s best for the consumer to trying to find out what the consumer actually wants. But even when they feign interest, things can go wrong if it’s not genuine or thorough enough.
Some instances are downright hilarious, like McDonald’s 2005 campaign, where it tried to emulate the youth hip lingo only for it to backfire horribly. Knowing that a certain group (young people) like something (their own jargon) doesn’t mean that the group will like any version of it, especially if it doesn’t fit or sounds like your dad trying to be “cool” and “with it.”
When McDonalds’ ad reads “Double Cheesburger? I’d hit it – I’m a dollar menu guy,” it becomes obviously painful they have no idea what they’re saying and they just try to sound knowledgeable (here you can find the “urban” definition of I’d hit it). And if there’s something worse than not fitting in, it has to be trying to fit in and failing. If you’re not with the cool kids, but you’re ok with that, you might still have a chance to earn respect.
If you’re not with the cool kids, but you’re desperate to get in, that’s when you’re doomed. Superficiality is definitely a mark of poor research.
Some similar misunderstanding of how youth works plagued McDonalds several years later. They tried to make something happen and “go viral” when they started sharing success stories using the “McDStories” hashtag. This turned out to be another failure – not only in consumer understanding, but also in technology understanding. The “McDStories” hashtag was soon reappropriated by random dissatisfied customers who decided to share their own stories. Only their recollections weren’t nearly as nice and flattering as the company’s. This particular example is extremely prescient to SEO, because it highlights the dangers of trying to manufacture goodwill. Something in the level of awareness of consumers and the technology tools they have at hand makes rebellion and irony very likely reactions to trend setting from the outside.
B) No Context
Market research matters. And poor data leads to poor business decisions. But you probably know that already.
Even when you do research thoroughly, you can still wrong your customers by not asking the right questions.
The classic example here is that of New Coke.
It wasn’t that their research was poor in itself: they had evidence that strongly suggested the taste was the leading cause of their market decline. They also had 200,000 blind product taste tests where more than half of respondents preferred the taste of the New Coke over both the “classic” Coke and Pepsi. So all the science was rightfully pointing them towards introducing the New Coke. Which they eventually did, with disastrous results. There was public outcry and the company ended up giving up the new formula and reverting to the classic one.
So what went wrong? Was it the consequences of poor data? The same thing, really: they didn’t fully understand their customers (although in a slightly different way than McDonalds). Coke’s mistake was to treat their customers as living in a vaccum, as consumers of soda, as opposed to consumers of Coke, specifically.
Blind tests are a very good instrument to use in general – except when you don’t want your respondets to be blind to their options.
So try to think about things not just in terms of functionality, but also brand recognition and emotional attachment.
It might very well be that your website’s design is outdated or boring. And the research reveals that youngsters are usually attracted to shiny, slick things. But maybe your particular type of outdated and boring is what works for your users. Data governance and information management are highly important; yet, so is data profiling.
Try to keep a certain perspective on things and make sure you ask the right questions about your consumers.
C) No Match
Other times, it could be more of a mismatch problem between companies and customers. Inadequate market research is a major reason why business strategies fail and the source of poor data. If you want to develop your brand, one of the most important things that you should establish is a reliable marketing research system. Customers will buy or not a product for a certain reason. And it is essential for the future of your company to understand what this reason is.
What drives your customers? What sends them away? What makes them continue with your services?
And the answer to all of these is just under your nose. Your sales and support team. They are a goldmine. Just think about it; what better way to find exactly what your users want if not from them directly, with no intermediates, no fancy feedbacks, just straightforward accurate data you can make use of. It depends, of course, on how you “master” data and how you make use of it. If, for instance, someone is asking you for a new feature you don’t have; yes, it’s frustrating as you might get dozens of similar mails. Yet, make use of them, exploit them and win customers (and therefore money). Take a look at the screenshot below and notice all the missed opportunities.
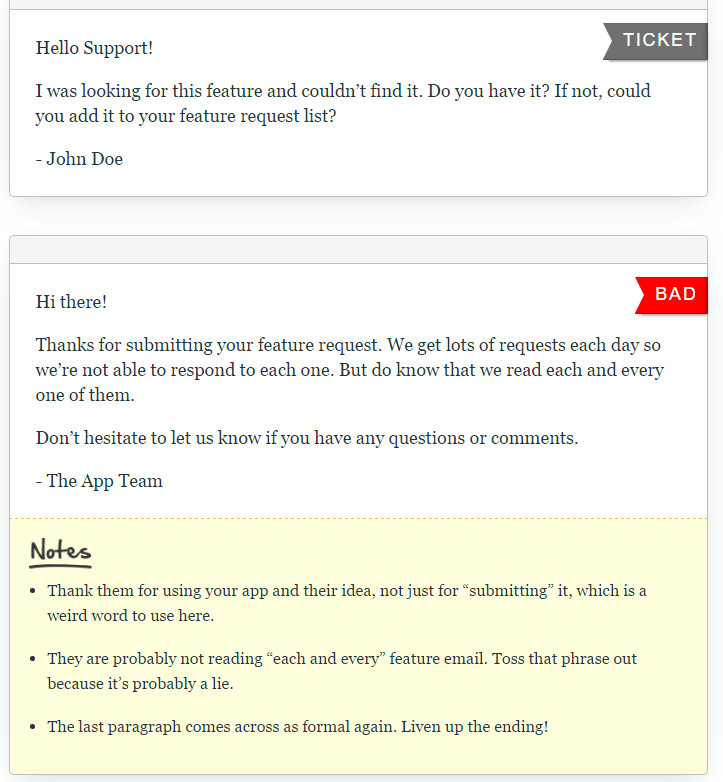
Source: helpscout.net
Through all its efforts, a company/business/website is trying to find a way to best reach its audience. Some efforts are sort of an “indirect” contact with the public through online content but there are also some others which are in direct contact. A discussion with the sales and support team might be a great opportunity for you to better understand your audience’s needs or challenges and adapt your strategy accordingly.
If you’ve never thought of your support team as a research tool, then you might be missing a lot.
Yet, let me present you what a mismatch between companies and customers can lead to through an interesting case study from the brand association area. Only by looking at the screenshot below you can forsee the association issues between Tesco products and a fine wine production line.
Yalumba, an Australian wine company was selling well into the UK through Tesco and relying heavily on their association with that supermarket chain, confident of their success because of the successful association. However, they began to be squeezed on margins, so had a close look at what was happening with Tesco, and were astounded at what they found.
They discovered that their brand was being marketed by Tesco as a commodity – offered on promotion and regarded by consumers as a good wine, but nothing special because it was generally offered at a special price. Their market research discovered that their brand was not considered good enough for special occasions because it was a Tesco promotional wine. Great they did a market research after all yet, a bit too late.
It was not the consumers’ wine of choice, and there was little connection between the consumer and the brand. This is a classic commodity trap, causing margin pressure, and not sustainable on the longer term. The company had created this problem of a commodity trap caused by poor research and a link between brand loyalty and promotions that led to an expectation of lower price and hence, ultimately, unsustainable low margins for Yalumba.
2. Faulty Methodology Being Used to Collect Digital Marketing Data
On this chapter we are going to tackle the loaded choices and questions issues but until then we are going to talk a bit about how a faulty methodology can lead you to a wrong track. And it doesn’t have to be on a large scale but even on a minimal research that you are doing. Let’s say that we are interested in the “restaurants” niche in Brasil. And we want to see everything that is out there on the web written on “restaurants” in the South American country. I use Brand Mentions for that and as you can see in the image below.
That’s indeed an impressive number of mentions. Yet, the initial search was done using the word I was targeting in english, “restaurants”. Will the search of the same word but in Portugese would make a difference? It sure does. A lot of difference. Therefore, even when doing a simple search you should always keep in mind the methodology if you want accurate data.
Below you will find some very interesting case studies based on some common faulty methodology.
A) Loaded Questions
How you phrase the questions and how much (or how little) information you decide to provide your respondents can make all the difference.
For instance, in the image below, which line is longer? If you bear with us until the end of the chapter you will find out the answer if you don’t know it already.
Giving them too little information can obviously skew their ability to give a useful answer. But it turns out that giving too much information can have the same effect. In his post on market research failures, Philip Graves provides two excellent examples of this phenomenon at work:
1. One is of a bank-related survey, where people are first asked if they have changed their bank lately and second if they are happy with their bank. While this line of questioning doesn’t necessarily provide unwanted information, it does create it implicitly in the mind of the respondent.
Just imagine: if you’ve answered “No” to the question about changing banks, once you get the second question, your brain is tempted to give an answer that is coherent with the answer to the first question. You can’t answer “No” to the second question, or else your answer to the first question would seem weird in retrospect. Have you changed your bank lately? No! Are you happy with your bank? The “Yes” comes automatically almost. Why would you not change your bank if you’re unhappy?
But of course, that’s not something you can do on the spot. What you can do on the spot, however, is find an answer that is consistent with the previous one, which, in this case, is “Yes.” You haven’t changed your bank because your are happy with it. There doesn’t need to be a causal link between the two, but the two answers are easier to accept if there is. We like order, symmetry and harmony.
When conducting a research, make sure the questions are consistent, logical and easy to understand.
2. The other example in Graves’ article is more glaring. In a survey that asked “As the world’s highest paid cabin staff, should BA cabin crew go on strike?” 97% of respondents said “No.” To nobody’s surprise, we should add. This a classic example of a loaded question. The way it is worded makes it very unlikely that someone might say “Yes,” since we’re talking about the world’s highest paid cabin staff. In fact, the mechanism at work here is very similar to the previous one – it’s just more explicit. Our brains find it hard to hold two opposing views, so we try to adjust one of them to fit the other.
It’s like asking: being the best tool in the digital marketing with 80% success rate, do you think that the pros should choose us?
In this case, the two implicit views at work are “people who are well-paid should be happy with their work” and “people who are happy with their work shouldn’t go on strikes.” Or, to further reduce them to equation-like mantras, “money = satisfaction” and “satisfaction ⇏ manifesting dissatisfaction.”
This kind of thinking – of which we are often not aware – is something that Nobel laureate Daniel Kaheman talks about at length in his “Thinking, Fast and Slow” book. It’s easier and faster to think in terms of simple equations and binary relationships between things, so we usually do. We are also prone to take the path of least resistance, so depending on how the question is phrased, we are more likely to give the answer that requires the least mental effort.
Just like in the case of the lines from the image above; even if you know, at a cognitive level that the lines are equal, you still have the tendency to consider the first one as being longer.
Phrasing questions in a survey is such hard work.
B) Loaded Choices
Loaded questions are just one example of asking the right questions in the wrong way.
An inherent bias in the language you use can also have a significant effect on the answers you get.
For instance, there is a huge difference between asking people “How satisfied are you?” and “How dissatisfied are you?” even when the answer options are exactly the same (for instance: “Very safisfied,” “Satisfied,” “Rather satisfied,” “Rather dissatisfied,” “Dissatisfied,” “Very dissatisfied”). Priming can have a very strong effect even if people don’t realize they are susceptible to it and even when they downright deny it.
But getting the question right isn’t enough – you have to also get the choices right. Have open-ended questions and you might get answers that aren’t comparable and end up not being useful.
Have closed-ended questions and you might end up not including some very relevant answers, which in turn will force respondents to provide answers that do not represent them.
Even if you go for a mixed approach, you can still mess up by not making the choices comparable or symmetrical at both ends.
Let’s say that you are asked about your assessment of a mailing automation service and the options were: “Excellent,” “Very good,” “Good,” Very poor,” “Disastrous.” If you were just mildly dissatisfied with the service you received, you’re not likely to signal that because unless you’re up in arms about it, there’s no answer that fits your level of dissatisfaction. And even if you were seriously dissatisfied, you’d more likely go for “Very poor” than for anything else, because “disastrous” is generally a very rare occurrence. Moreover, the fact that there are 3 positive options and only 2 negative ones makes the least positive option (which is still quite positive) appear as more of a middle-ground than it should. So, if you’re just slightly dissatisfied, you are more likely to keep it to yourself and choose “Good” rather than choose “Very poor.” This is, obviously, an exaggerated example, but there are plenty, more subtle – but equally problematic – research design flaws out there.
3. Data That Cannot Be Translated Into Conversion
Here we are talking about useless data actually. Not quality issues, inaccurate data, business process, data managements, cost associated or process quality issues like we’ve discussed so far. We’ve concluded by now that the quality of online data is a major concern—not only for the market research industry but also for business leaders who rely on accurate data to make decisions.
Sometimes companies are so focused on the mousetrap they tend to lose touch with the emotions and needs of the people they actually work for: consumers.
Let’s say for instance that you are planning on boosting your website through SEO. And one day you realize that you have around 3 million links. That’s amazing, right? A few more and you are catching Microsoft’s link profile. Yet, is there anything you can do with this info alone? The 3 million links? Not really. You need to correlate it with the number of referring domains (which in our case is incredibly low reported to the high amount of links), the link influence, their naturalness, etc. Otherwise, there is no much use in having just the number of links. You cannot set up a strategy based on it nor take a digital marketing decision.
Yet, these things happen to the big guys as well. Let’s take Google Glasses for instance. Not even 2 years have passed from the great launch and the producers announced that they are discontinuing the product. Didn’t Google have the means to conduct a proper research? Of course they did. Then what happened?
What did they overlook? Everything, some would say. Well, not quite everything but it’s clear that they made use of the data they had but not in an efficient way (we can say that these are still consequences of poor data). After researches, the producer came up with one conclusion: if high tech can be shrunk to fit in the hand, as with smartphones and tablets, and have such a great success, then why not shrunk technology to be close to a part of the human body? And their market research sample seemed thrilled about the idea. This was the info they’ve provided to the company. The product is created, it gets launch and no glory at all. Even more, it unceremoniously fails within months. So what happened? Was it the consequences of poor data? The producers made their potential customers’ dreams come true; why did they not sell?
Privacy and price concerns alienated much of the public—and the fact Google released early copies only to rich geeks in the west coast, thereby branding the product as elitist instead of the “affordable luxury” philosophy. Even if they had a lot of data, it was quite useless. According to CNN, before launch, Google didn’t anticipate that few would want to pay (or could afford) $1,500 to parade in public with a device slung around their eyes that others might perceive as either a threat to others’ privacy or a pretentious geeky gadget.
“Google’s fast retreat exposes the most fundamental sin that companies make with the “build it and they will come” approach. It’s a process that tech companies rely on, referred to as public beta testing.”
There are blind spots in marketing research that are costly and variables that no market research studies can predict.
Conclusion
Bad data can lead to bad analysis, which can lead to bad business decisions, some really bad revenue and not only. The first step is made. If you are aware of poor data quality impact today, it will not affect your business tomorrow. One should always be aware that bad data has long time consequences not only on your overall data management but also on all your future business decisions.

Leave a Reply!